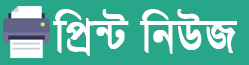
These strategies embrace strategies similar to re-ranking, threshold adjustment, and fairness-aware ensemble strategies. Re-ranking entails reordering the model’s predictions to ensure honest illustration, while threshold adjustment modifies the decision threshold for different groups to attain equitable outcomes. Fairness-aware ensemble methods mix multiple fashions to supply a ultimate prediction that balances accuracy and fairness. Adequately representing underrepresented groups is particularly essential because these groups might need traditionally been marginalized or subjected to systemic biases. By including these groups’ experiences and perspectives within the training data, bias-aware algorithms might help handle these inequalities and promote fair decision-making. AI governance frameworks guarantee transparency, accountability, and equity in AI decision-making.
Algorithms skilled on incomplete or skewed data prioritize sure demographics, influencing who sees high-value merchandise or unique deals. This article will discover AI bias and why assuaging it must be a high business precedence. We’ll additionally look at some main principles, the various varieties of AI bias, and the way they impact trendy business. The Brookings Establishment is committed to high quality, independence, and influence.We are supported by a diverse array of funders.
Join Us Now And Get Your Free Copy Of “software Development Cost Estimation”!
By integrating moral issues into AI improvement, we will create technologies that respect human rights, uphold societal values, and promote a extra just and equitable world. Addressing bias in AI algorithms is a critical step in the direction of constructing accountable and reliable AI technologies. It is imperative that we prioritize the development of unbiased and responsible AI to ensure a good and just future. This may involve adjusting the language used, refining the info units, or modifying the underlying algorithms. By constantly reviewing and updating your AI methods based mostly on feedback, you’ll be better geared up to deal with biases as they emerge and preserve fair and equitable AI-driven solutions. Synthetic intelligence (AI) has emerged as a strong what is the role of a bias in ai models device that has the potential to revolutionize numerous elements of our lives.
When the individual in the photograph was a white man, the software was accurate 99 % of the time at figuring out the particular person as male. Nevertheless, whereas enhancing mannequin generalizability, federated learning inherently limits staff entry for knowledge pre-processing or quality assurance duties, limiting its appropriateness for specific purposes. Implicit bias occurs when unconscious attitudes or stereotypes a few https://www.globalcloudteam.com/ person’s or group’s characteristics, corresponding to start sex, gender identity, race, ethnicity, age, and socioeconomic standing, turn into embedded in how individuals behave or make selections.
Organizations should be sure that their AI systems adhere to those regulations to avoid authorized repercussions and maintain user trust. AI methods typically depend on private and sensitive information, such as well being records, financial info, and consumer habits. Safeguarding this data is essential to protect individuals’ privacy rights and stop potential misuse or information breaches. The power of AI lies in its capability to process huge amounts of information to derive significant insights and predictions. Nevertheless, the abundance of knowledge required to gasoline AI techniques raises significant data privateness and safety concerns. In this section, we will delve into the challenges and finest practices for safeguarding person JavaScript information and ensuring compliance with privacy rules within the context of AI.
- This deployment bias has been shown to result in the dismissal of appropriate important warnings, with potential risk of affected person harm47,49.
- Moreover, biased AI algorithms can perpetuate present stereotypes, reinforcing harmful prejudices and resulting in inaccurate assessments of people.
- In this section, we’ll discover the significance of transparency and explainability in AI systems and their influence on person belief, accountability, and moral deployment.
- AI already impacts people’s lives in the actual world, making mitigating AI bias even more important.
- Representation steadiness is essential; your training knowledge should reflect the range of the inhabitants your AI will serve.
- This means looking beyond easily accessible information and making a concerted effort to include underrepresented voices.
Understanding Bias In Ai And Its Implications
One method to continuous monitoring is conducting common audits of an algorithm’s efficiency, analyzing the outcomes of algorithmic decisions, and assessing whether or not any disparities or biases exist across totally different demographic teams. By evaluating an AI algorithm’s outcomes and figuring out potential discrepancies, organizations can take proactive steps to rectify biases and enhance the general equity of the system. This may contain analyzing output information for patterns that might point out unfair remedy of sure groups. When points are discovered, it’s crucial to address them promptly and transparently. Bear In Mind, equity and inclusivity aren’t simply ethical concerns – they’re important for constructing belief together with your clients and sustaining a positive reputation in the lengthy run.
Additional, human biases can be bolstered and perpetuated without the user’s knowledge. For example, African-Americans who’re primarily the target for high-interest credit card choices might find themselves clicking on this sort of advert without realizing that they’ll continue to receive such predatory on-line recommendations. In this and different circumstances, the algorithm may never accumulate counter-factual advert ideas (e.g., lower-interest credit score options) that the consumer could presumably be eligible for and prefer. Thus, it’s important for algorithm designers and operators to watch for such potential adverse suggestions loops that trigger an algorithm to become more and more biased over time. As Synthetic Intelligence (AI) becomes more and more pervasive in our daily lives, concerns about AI bias have grown exponentially. AI bias refers to the unfair outcomes or discriminatory behavior exhibited by AI techniques, typically ensuing from biased information or algorithms.
This perception helps you determine and handle potential biases in the system’s outputs. To create effective suggestions loops, think about implementing common evaluate processes the place you analyze the AI’s outputs for signs of bias. Encourage customers to report any regarding outcomes, and establish clear channels for them to take action. It’s vital to actively seek numerous views to ensure you’re capturing a variety of potential biases.
When the training information used to construct the algorithms contains inherent biases or reflects societal preconceptions, bias can exist in AI techniques. Biases can also be created in the course of the algorithmic design and implementation processes. Involving people from totally different backgrounds, with totally different viewpoints and experiences, aids within the discovery and correction of biases through the improvement process. Vast groups usually have a tendency to spot potential biases, question assumptions, and create more inclusive AI systems that represent the vast spectrum of individuals they serve.
Be A Part Of us in unraveling the complexities of bias mitigation in generative AI and uncover how we will create extra equitable and dependable AI systems. Some decisions might be greatest served by algorithms and different AI tools, whereas others might have considerate consideration before computer models are designed. Further, testing and evaluation of certain algorithms may even determine, and, at best, mitigate discriminatory outcomes. For operators of algorithms seeking to reduce the danger and issues of dangerous outcomes for consumers, the promotion and use of the mitigation proposals can create a pathway towards algorithmic fairness, even if fairness isn’t absolutely realized. Incorporating DEI principles have to be perceived as an essential precedence, particularly given an absence of representation in current regulatory tips for AI applications50,eighty three.
Involve numerous stakeholders in the course of, including ethicists, authorized experts, and representatives from totally different departments. This multidisciplinary method will assist determine potential blind spots and create a more thorough framework. Regularly review and update your ethical pointers to keep pace with evolving AI technologies and emerging moral challenges.